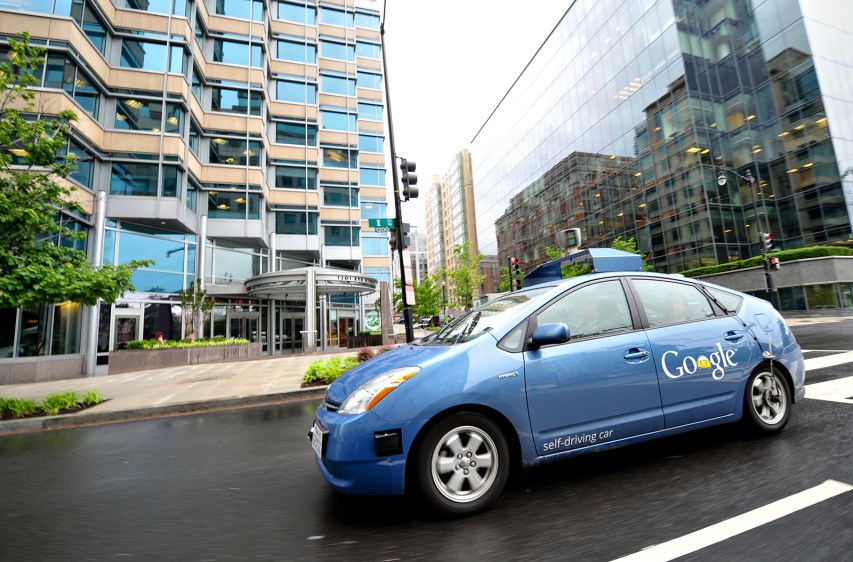
That Street View car driving down the street is snapping immersive images for Google Maps, but that visual data can be used to map much more—socioeconomic trends like crime and rates and household income to voting patterns.
If that Mars Rover-like camera records more pick-up trucks than sedans during a 15-minute drive through a city, for example, a team of researchers can use that information to determine the area is more likely to vote Republican during the next Presidential election. This prognostication isn’t some dark art, but the product of machine learning and computer vision—a computer science field that’s exactly what it sounds like, teaching computers to “see” patterns.
Timnit Gebru and a team of researchers at Stanford Vision Lab matched the results from their algorithm, created from data points given by Google Street View, and found a strong enough correlation to make precise predictions across voter precincts, comprising about 1,000 people each. According to Technology Review, techniques like this have “great potential to provide almost real-time monitoring of changes in the population.”
Together, machine learning and computer vision have contributed to the computer equivalent of the Enlightenment in the last decade. During this time, computers have gone from seeing to being used to help humans see their world better. Largely, the rapid advance of this field is due to the work of pioneers like Gebru and Fei-Fei Li at Stanford Vision Lab.
A few years ago, Dr. Fei-Fei launched ImageNet, a crowdsourced platform of 14 million photos with annotations that help computers learn to see. It was basically a proof-of-concept for using machine learning to help power computer vision. Together, the technologies help computers mature in their object recognition capabilities and give better context.
Today, computer vision is at the forefront of some of the most cutting-edge technologies. Perhaps no industry has been shaped more rapidly by these applications than e-commerce.
Social media giant Pinterest—which serves up lifestyle hacks, style guides, and recipes on user-generated “boards”—recently unveiled a new feature. Lens lets people point their phone at an object, like an Eames chair, and identify it as well generate suggestions for similar products in that.
The feature uses computer vision to find the “most visually similar objects in billions of images in a fraction of a second” and then employs machine learning to “map those objects to the original image and return scenes containing the similar objects,” Andrew Zhai, Pinterest tech lead, explains in a blog post.
Magnus, an art-identifying app, works in a similar way. Over four years, the team behind the Magnus developed a catalog over 10 million pieces of art tagged with the artist’s name, its price, and points of sale too. “We make art more accessible,” Magnus Resch told RealClearLife. “Instead of just taking a picture of art, you get something out of it.” Although the app has a matching rate of 70 percent, Resch says the 100,000 images added each month is helping to make the app’s technology more precise.
Computer vision is already contributing to the development of tomorrow’s revolutionary technology—including self-driving cars. SkinVision, an app available outside the U.S. for now, gives melanoma diagnoses from a smartphone. Billboards in Moscow already deliver ads targeted to specific drivers based on the car they’re driving, Technology Review reports.
Gebru points out this same context provided by machine learning, also creates limitations to futuristic technologies.
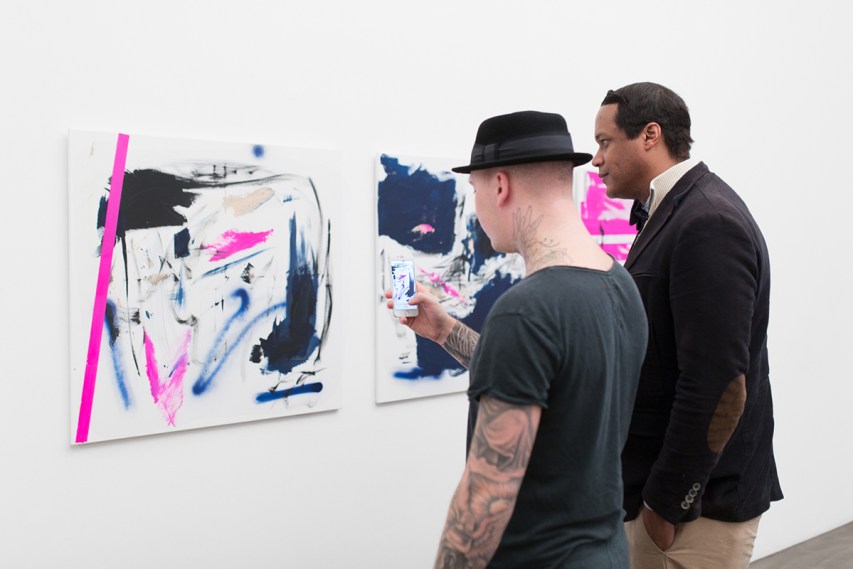
While it’s something that can be overcome, the learning curve for what’s called domain adaptation (applying the learning from one set of data to another) is far steeper for a computer when more variables enter the equation and the stakes are raised. It’s evidenced by Magnus’ match rate of 70 percent; and Pinterest’s Lens and Magnus still can’t recognize objects perfectly in every single environment. If they did, Gebru says she “would be pretty amazed.”
These applications of computer vision will get better with time—much like the predictions of its other applications. “The thing that gets the most hype right now is not necessarily the thing that five years from now you look back and say, ‘this is so useful,’” Gebru explains.
For now, though, we’ll be placated by our Snapchat filters and our endless sneaker choices.
—RealClearLife
This article was featured in the InsideHook newsletter. Sign up now.